The Essential Role of Image Datasets for Object Detection in Business
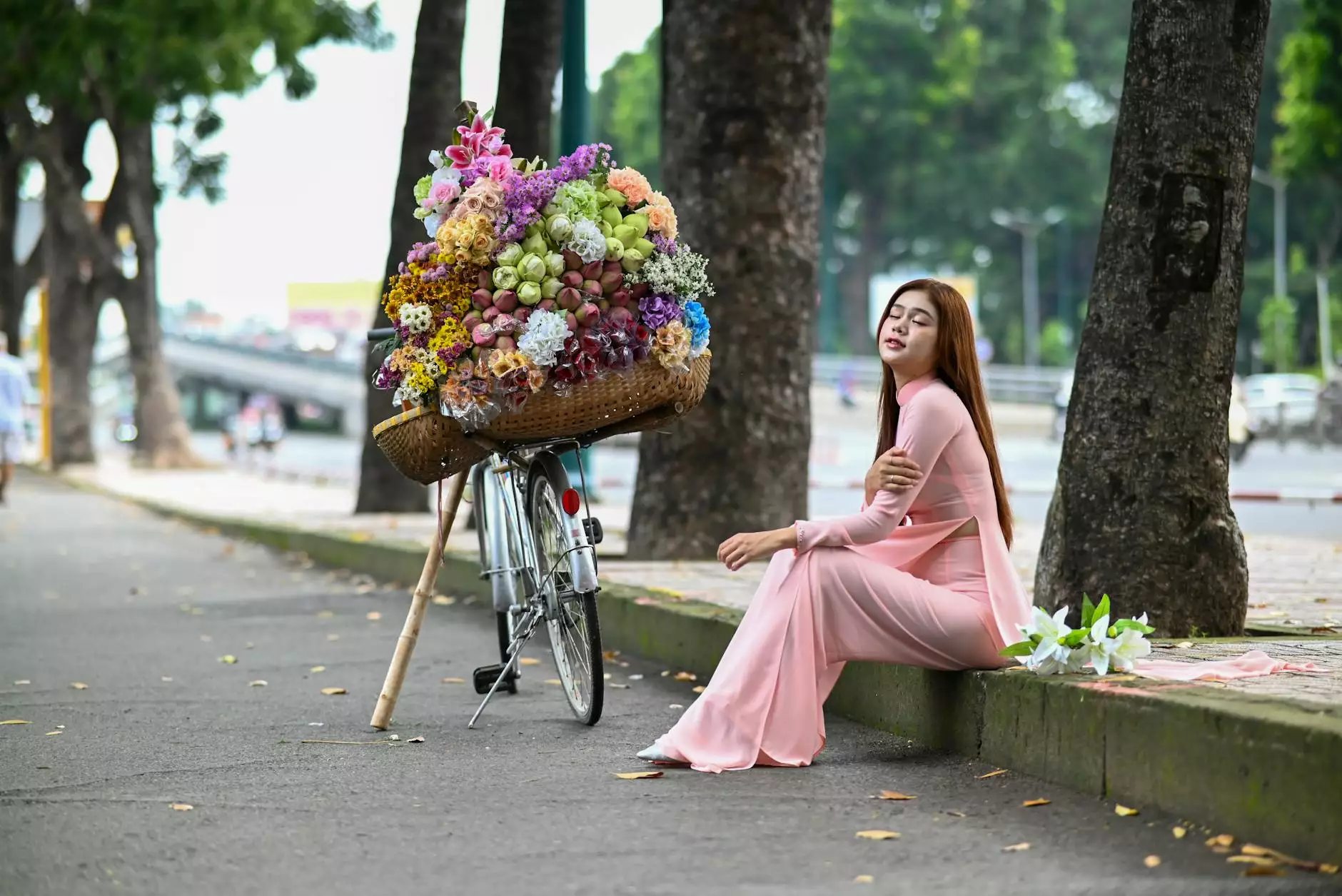
In the rapidly evolving landscape of technology, businesses are continuously searching for ways to leverage artificial intelligence (AI) and machine learning (ML) to enhance their operations. At the heart of many AI applications, particularly in computer vision, lie image datasets for object detection. This article explores the critical role these datasets play in software development and how they can propel businesses forward.
What Are Image Datasets for Object Detection?
Image datasets for object detection are collections of labeled images used to train algorithms to recognize and localize objects within images. These datasets encompass a variety of images containing different objects, annotated with specific labels and bounding boxes that indicate the location of each object in the image. The ability to accurately detect and classify objects in images is a fundamental requirement in numerous applications, from autonomous vehicles to facial recognition software.
Why Are Image Datasets Important?
Image datasets are crucial for several reasons:
- Training AI Models: AI models require vast amounts of data to learn and improve. Datasets provide the necessary samples for these models.
- Benchmarking Performance: Researchers and developers need a standard dataset to evaluate the effectiveness of their models. This allows for consistent comparisons and improvements.
- Sparking Innovation: With the right datasets, businesses can innovate and create new applications that harness the power of object detection.
Types of Image Datasets for Object Detection
Understanding the different types of image datasets is essential for developers and businesses looking to implement object detection capabilities:
1. Public Datasets
Many public datasets are available, created by universities, research organizations, and companies. Examples include:
- COCO (Common Objects in Context): A large-scale dataset designed for object detection, segmentation, and captioning tasks.
- PASCAL VOC: A well-known dataset used in object detection competitions featuring a variety of categories.
- ImageNet: Although primarily known for image classification, it includes categories that support object detection tasks.
2. Private Datasets
Many companies choose to create their own datasets specific to their needs. This option ensures that the training data is aligned closely with the business requirements and the unique entities the model needs to recognize.
Key Considerations When Creating Image Datasets for Object Detection
When developing an image dataset for object detection, several best practices should be followed to ensure the dataset is effective:
1. Annotation Quality
The quality of the annotations in your dataset directly affects the performance of your object detection model. Ensure that:
- The bounding boxes accurately fit the objects.
- Labels are consistent and relevant.
- Various angles and lighting conditions are represented.
2. Diversity of Images
A diverse dataset is crucial for training robust models. Include a wide variety of backgrounds, object sizes, and occlusions to cover real-world scenarios.
3. Sufficient Sample Size
Having an adequate number of images for each class is essential. The more diverse and numerous the samples, the better your model can generalize to new images.
The Process of Building an Image Dataset for Object Detection
Building an image dataset requires a systematic approach:
1. Data Collection
Collect images from various sources, such as open-source repositories, company archives, or new photography, ensuring you have the right to use these images legally.
2. Data Labeling
Label your images using tools designed for annotation, such as LabelImg or VoTT. This step is crucial, as the accuracy of the labels directly impacts model performance.
3. Data Augmentation
To enhance the dataset, apply data augmentation techniques that artificially expand the dataset. Techniques include:
- Flipping
- Rotating
- Color variations
- Cropping
4. Quality Assurance
Conduct rigorous quality assurance checks to ensure that your dataset complies with the previously mentioned standards of annotation and diversity.
Utilizing Image Datasets for Business Gains
The implementation of object detection technologies, powered by robust image datasets, can lead to significant business benefits:
1. Enhanced Operational Efficiency
By automating object detection processes, businesses can streamline operations, reduce labor costs, and increase accuracy in various tasks, such as inventory management.
2. Improved Customer Experiences
Companies can personalize customer experiences by employing object detection in retail settings, enabling smart recommendations based on visual cues.
3. Innovative Product Development
With advanced object detection capabilities, organizations can explore new avenues for product development, including advanced robotics and automated surveillance systems.
The Future of Image Datasets in Object Detection
As technology advances, the future of image datasets for object detection appears promising. We can expect:
1. Increased Collaboration
As more organizations recognize the importance of dataset sharing, we will see increased collaboration, leading to richer datasets that benefit all community members.
2. Automating Dataset Creation
With AI-driven annotation tools on the horizon, the time-consuming process of creating datasets may soon become automated, significantly reducing upfront costs and time investment.
3. Expansion of Use Cases
The application of object detection will continue to expand across industries, including healthcare, security, and agriculture, driving innovation and efficiency in unexpected areas.
Conclusion
In conclusion, image datasets for object detection are a powerful resource for businesses looking to enhance their software development and operational efficiency. By investing in quality datasets, companies can not only accelerate their AI projects but also gain a competitive edge in the market. The landscape of AI and ML is vast and continuously evolving, making it imperative for businesses to stay ahead through comprehensive data strategies.
For enterprises aiming to innovate with software solutions, understanding this intersection of technology and business is crucial. By prioritizing the generation, quality, and utilization of image datasets, organizations can harness the full potential of object detection technology.