Revolutionizing Machine Learning with an Image Annotation Tool
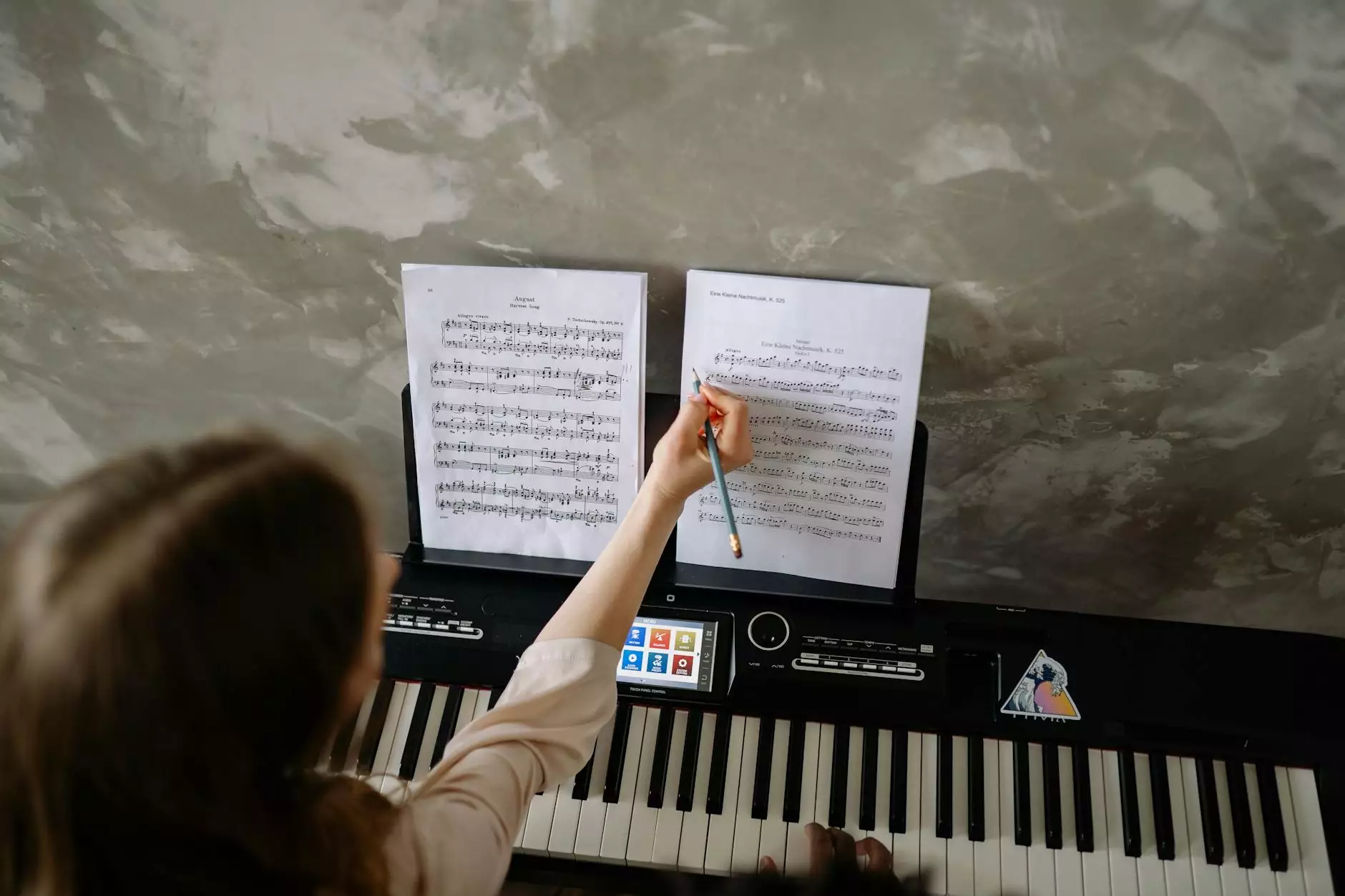
In the evolving landscape of technology, one of the most transformative breakthroughs has been in the field of machine learning. As businesses strive to harness the power of artificial intelligence, the necessity for effective data labeling becomes increasingly apparent. An image annotation tool for machine learning plays a crucial role in this process, serving as the backbone for training sophisticated algorithms. This article explores the significance, benefits, and intricacies of image annotation tools, while positioning Keylabs.ai as the leader in this domain.
Understanding Image Annotation
At its core, image annotation involves the process of labeling images to provide contextual information to machine learning models. This can include identifying objects, tagging, segmenting, and describing visual data. The task requires accuracy, detail, and a robust understanding of what information needs to be extracted from the images. As machine learning systems become more reliant on diverse datasets, the demand for precise annotation has surged.
The Role of Image Annotation in Machine Learning
The success of any machine learning model hinges upon the quality of the data it learns from. Here’s how image annotation contributes to this success:
- Improved Predictive Accuracy: By providing detailed annotations, models learn to recognize patterns with greater accuracy.
- Enhanced Model Training: Well-annotated images help in refining algorithms, leading to better performance during inference.
- Diversity of Data: Annotated datasets can represent a variety of scenarios, which is critical for training robust models in real-world applications.
The Benefits of Using an Image Annotation Tool
As organizations navigate the complexities of deploying machine learning models, the benefits of utilizing an image annotation tool for machine learning become evident:
- Efficiency: Automated tools significantly speed up the annotation process, enabling businesses to scale their data pipelines.
- Cost-Effectiveness: By reducing the time spent on manual labor, companies save substantial resources in the long run.
- Collaboration: Advanced platforms facilitate teamwork among data scientists and annotators, fostering a more productive environment.
- Quality Control: Annotations can be easily reviewed and verified, ensuring high-quality data outputs.
Key Features of a High-End Annotation Tool
When selecting an annotation tool for image data, it's crucial to consider various features that enhance usability and functionality:
- User-Friendly Interface: A simple and intuitive design allows users to navigate efficiently and focus on their tasks.
- Multiple Annotation Types: Support for bounding boxes, segmentation, polygons, and keypoint annotations caters to a diverse range of use cases.
- Integration Capability: Seamless compatibility with existing data pipelines and AI frameworks ensures a smooth workflow.
- Collaboration Tools: Features such as comments, tagging, and progress tracking promote effective teamwork.
- Analytics and Reporting: Built-in analytics help track progress and annotate quality, allowing for continuous improvement.
How to Choose the Right Image Annotation Tool
Choosing the right data annotation platform is crucial for your machine learning projects. Here are guiding factors that should influence your decision:
1. Identify Your Requirements
Before diving into the search for an image annotation tool, outline your specific needs. Consider the following:
- The size of your dataset.
- The types of annotations required (bounding boxes, segmentation, etc.).
- Your budget constraints.
2. Evaluate Features and Functionality
Examine the features of potential tools as described above. Make sure they align with your requirements and enhance your team's productivity.
3. Consider User Support and Community
Look for platforms that provide robust customer support and an active user community. This aspect can significantly ease troubleshooting and foster best practices.
4. Integration with Existing Systems
Ensure that the image annotation tool can integrate with your existing AI frameworks and data infrastructure, facilitating a seamless workflow.
5. Request a Demo
Most reputable annotation tool providers offer demos or trials. Utilize these offerings to assess the usability of the tool and determine if it truly meets your needs.
Case Studies: Success Stories with Image Annotation Tools
To illustrate the profound impact of an image annotation tool for machine learning, let’s explore some successful implementations in various industries:
1. Autonomous Vehicles
Companies in the automotive sector have leveraged image annotation tools to train their autonomous driving systems. By accurately annotating images of roads, traffic signs, and pedestrians, these models have dramatically improved in their ability to navigate complex environments, ultimately enhancing the safety and reliability of self-driving cars.
2. Healthcare Imaging
The healthcare industry has utilized image annotation to aid in diagnosing conditions through medical imaging. By annotating scans, radiologists can train models to detect anomalies, which can lead to earlier diagnoses and better patient outcomes.
3. E-commerce and Retail
E-commerce platforms employ image annotation to automate product categorization and inventory management. By effectively labeling products in images, these systems can recommend similar items, refine search results, and enhance the overall shopping experience.
The Future of Image Annotation Tools
As technology continues to advance, the future of image annotation tools looks promising. Here are some emerging trends to watch:
- AI-Assisted Annotation: The integration of AI to assist in the annotation process is set to revolutionize data labeling, significantly reducing manual effort and time.
- Real-Time Annotation: With improvements in processing power, real-time annotation capabilities are becoming more feasible, enhancing workflows in dynamic environments.
- Customization: Future tools may offer greater customization options to tailor the annotation processes to specific industry needs.
- Enhanced Collaboration: With the rise of remote work, tools that enable efficient collaboration across global teams will be instrumental in success.
Conclusion
In conclusion, the reliance on an image annotation tool for machine learning cannot be overstated. As businesses navigate through vast arrays of data, the ability to annotate images accurately and efficiently significantly impacts the performance of machine learning models. By leveraging cutting-edge technology, organizations can transform their data into actionable insights and drive innovation in their respective fields. Keylabs.ai stands at the forefront of this movement, providing robust annotation solutions tailored to meet the diverse needs of businesses around the globe.